The Importance of Data Labeling in Modern Business Strategies
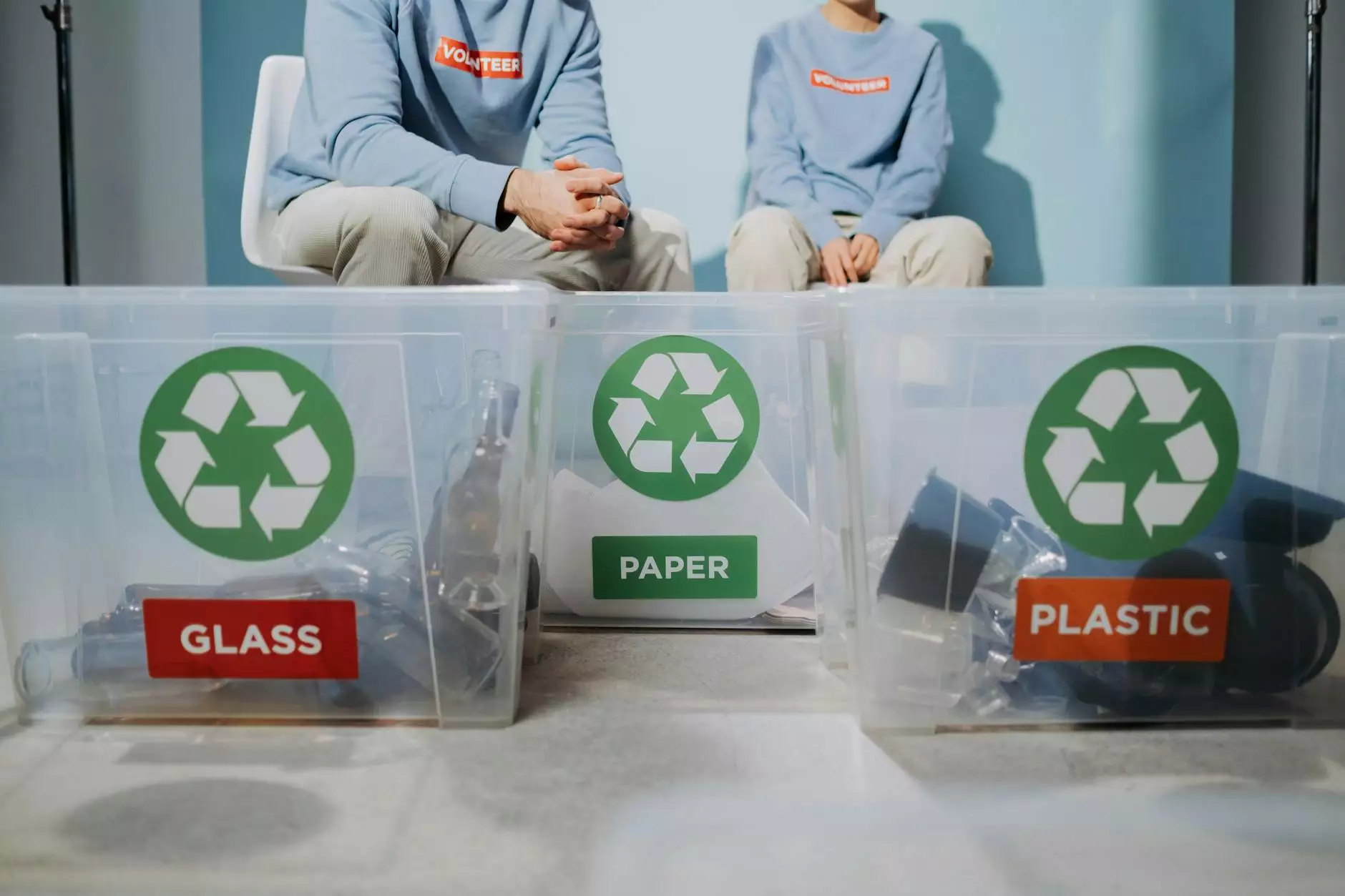
In today's rapidly evolving technological landscape, data labeling has emerged as a critical component in the development of artificial intelligence (AI) and machine learning (ML) applications. As businesses strive to harness the power of AI to drive innovation and gain competitive advantages, understanding the pivotal role of data labeling becomes essential. This article delves deep into data labeling, its applications, benefits, and how companies like KeyLabs.ai are transforming this process through advanced data annotation tools and platforms.
Understanding Data Labeling
At its core, data labeling refers to the process of annotating or tagging data with relevant information. This includes adding metadata to both structured and unstructured data such as images, text, videos, and audio files. The goal is to effectively train machine learning models so that they can interpret and analyze data accurately. Labeling can take various forms, including:
- Image Annotation: Identifying objects within images, such as cars, people, or animals.
- Text Annotation: Marking up text data to identify sentiments, entities, or topics.
- Audio Annotation: Transcribing spoken words or labeling sounds in an audio file.
- Video Annotation: Tagging specific frames in videos for actions, objects, or events.
The Significance of Data Labeling in Machine Learning
Machine learning algorithms learn from data. Without accurately labeled data, these algorithms cannot make correct predictions or classifications. Here’s why data labeling is fundamental for businesses aiming to leverage machine learning:
1. Quality Data Drives Quality Insights
The saying "garbage in, garbage out" rings especially true in the realm of AI. When data is poorly labeled, it can lead to inaccurate predictions and insights. By employing rigorous data labeling techniques, businesses ensure that the data fed into their machine learning models is both accurate and representative, leading to better overall performance.
2. Enhanced Model Performance
Machine learning models rely heavily on the quality of the training data. Well-labeled data enhances the precision of these models, allowing them to generalize better to unseen data. Consequently, this can result in:
- Increased Accuracy: More precise predictions based on the labeled data.
- Reduced Bias: Ensuring diversity in the training set can minimize biases present in AI applications.
- Improved User Satisfaction: Higher accuracy leads to better user experiences and trust in AI systems.
3. Scalability and Efficiency
As businesses grow, so does the volume of data they collect. Manual labeling processes can become bottlenecks. Implementing automated data labeling solutions can massively improve efficiency, enabling organizations to scale their operations without sacrificing quality. This is where platforms like KeyLabs.ai come into play, providing cutting-edgedata annotation tools that leverage automation to streamline the labeling process.
Strategic Advantages of Data Labeling for Businesses
Investing in data labeling can provide businesses with several strategic advantages:
1. Gaining Competitive Edge
In the age of big data, businesses that adopt AI-driven practices gain substantial advantages over competitors. Effective data labeling allows these organizations to harness deeper insights from their data, enabling proactive decision-making and tailored customer experiences.
2. Cost Reduction
While the initial costs of setting up a robust data labeling process can be significant, the long-term savings are profound. Accurate models can significantly reduce the costs associated with errors, rework, and customer dissatisfaction. Moreover, being able to rapidly process and analyze vast datasets can lead to operational efficiencies that further drive down costs.
3. Enhanced Innovation
When businesses utilize accurately labeled data, they can experiment with new AI-driven approaches and strategies with greater confidence. This fosters a culture of innovation, propelling organizations toward new product developments, improved services, and enhanced operational practices.
Different Types of Data Annotation Tools
Various tools exist within the data annotation landscape, each suited to different needs and types of data. Here are some common types of data annotation tools that can significantly enhance the data labeling process:
- Image Annotation Tools: Tools that allow users to draw bounding boxes, polygons, or key points on images to label objects.
- Text Annotation Tools: Platforms that enable the marking of entities, sentiments, or categories within text.
- Video Annotation Tools: Tools that allow users to annotate moving videos frame by frame with specific labels and classifications.
- Audio Annotation Tools: Solutions that facilitate the transcription of speeches or sounds into labeled formats.
KeyLabs.ai: Revolutionizing Data Labeling
With the growing demand for efficient data annotation, KeyLabs.ai stands at the forefront of the industry. Their comprehensive data annotation platform provides businesses with seamless data labeling solutions tailored to meet the specific needs of various industries.
Robust Features of KeyLabs.ai Data Annotation Tools
KeyLabs.ai offers a suite of features designed to enhance the data labeling process:
- User-Friendly Interface: The platform boasts an intuitive interface that simplifies the labeling process for teams.
- Automation Capabilities: KeyLabs.ai automates repetitive tasks, significantly speeding up the annotation process while maintaining quality.
- Collaboration Tools: The platform enables teams to collaborate seamlessly, offering robust project management features and real-time communication.
- Quality Assurance: Integrated quality control checks ensure the data labeling is accurate and meets strict standards.
- Versatility: Capable of handling various data types, including images, text, audio, and video.
Industries Benefiting from KeyLabs.ai
Data labeling is vital across multiple industries, including:
- Healthcare: Labeling medical images for diagnostics and risk assessment.
- Finance: Analyzing transaction data for fraud detection and risk management.
- Retail: Enhancing customer experience through personalized marketing strategies.
- Autonomous Vehicles: Annotating sensory data for navigation and obstacle detection.
Conclusion
In conclusion, data labeling is not merely a technical necessity; it is a strategic imperative that can empower businesses to unlock the full potential of their data. By investing in robust data annotation tools like those offered by KeyLabs.ai, organizations can transform their data into actionable insights, driving innovation and maintaining a competitive edge in an increasingly data-driven world. As we continue to advance towards a future dominated by machine learning and AI, the importance of accurate and efficient data labeling will only continue to rise, solidifying its status as a cornerstone in the fabric of modern business strategy.